Harnessing the Power of Medical Datasets for Machine Learning in Healthcare
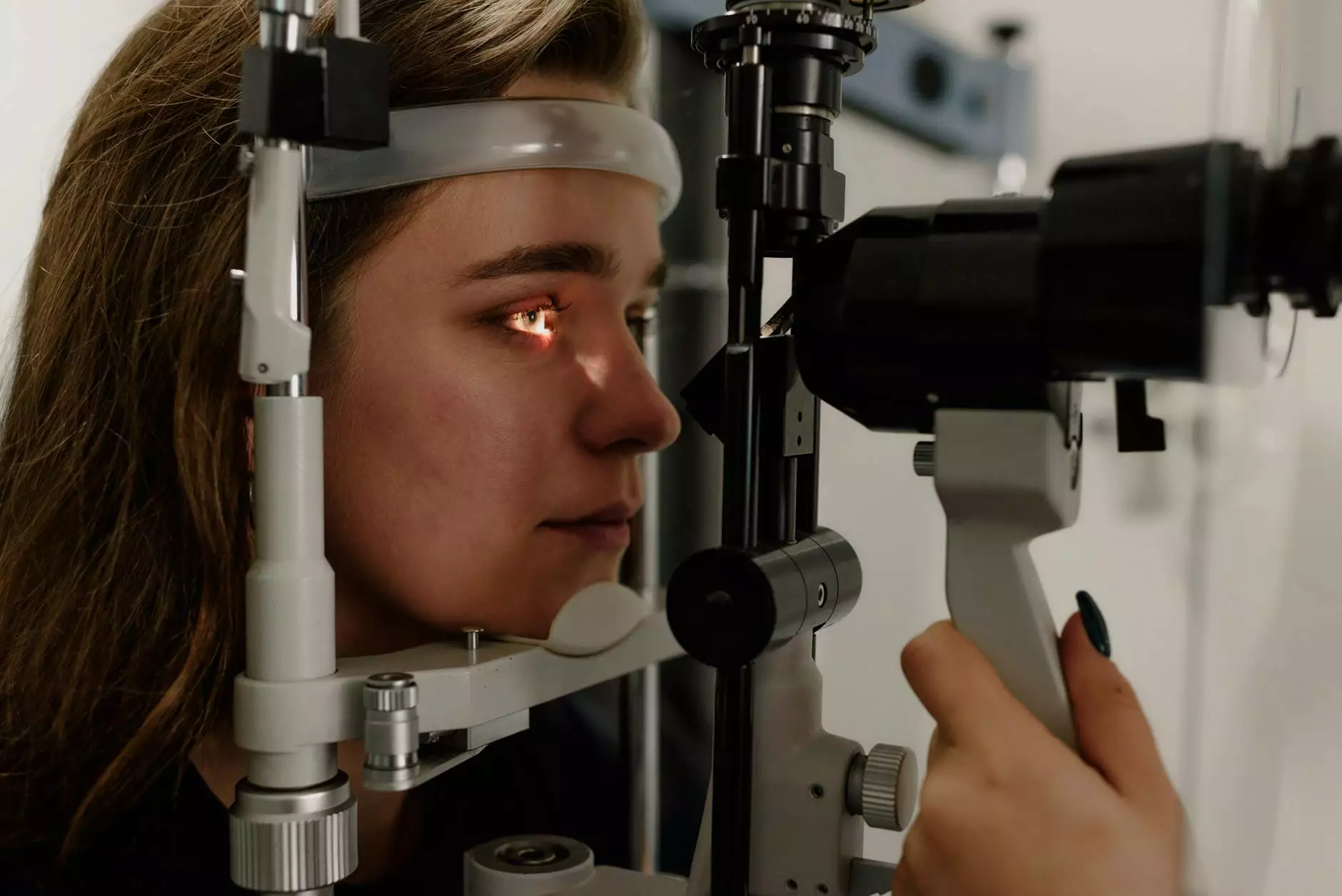
In the ever-evolving landscape of modern healthcare, the integration of technology and data has led to groundbreaking advancements. At the forefront of this change is the use of medical datasets for machine learning, which is revolutionizing how healthcare professionals approach diagnosis, treatment, and patient care. This article delves into the significance of medical datasets in machine learning, illustrating their transformative impact on the healthcare sector.
Understanding the Role of Datasets in Machine Learning
To comprehend the importance of medical datasets for machine learning, one must first grasp the fundamentals of machine learning itself. Machine learning is a subset of artificial intelligence (AI) that enables computers to learn from and make predictions based on data. In the context of healthcare, these datasets are crucial for training algorithms to recognize patterns, predict outcomes, and assist healthcare providers in making informed decisions.
Types of Medical Datasets
Medical datasets can vary significantly in structure and content. Here are some key categories:
- Electronic Health Records (EHRs): Comprehensive patient histories including diagnoses, treatments, and progress notes.
- Clinical Trials Data: Data collected from clinical trials, encompassing various patient demographics and treatment responses.
- Genomic Data: Information related to patients' genetic makeup, which can influence treatment plans.
- Imaging Data: Visual data such as X-rays, MRIs, and CT scans that can be analyzed for diagnostic purposes.
- Patient Surveys: Responses gathered from patients about their symptoms and treatment experiences.
Why Medical Datasets are Crucial for Machine Learning
The utility of medical datasets for machine learning cannot be overstated. Here are several reasons why they are indispensable in the field:
1. Improving Diagnostic Accuracy
Machine learning algorithms trained on extensive medical datasets can significantly enhance diagnostic accuracy. For example, algorithms can analyze imaging data to detect anomalies that a human eye might miss. This capability not only aids in quicker diagnoses but also reduces the likelihood of misdiagnosis.
2. Personalizing Treatment Plans
By leveraging medical datasets for machine learning, healthcare providers can create personalized treatment plans. For example, predictive analytics can determine how different patients might respond to specific treatments based on their historical data.
3. Predicting Patient Outcomes
Advanced machine learning models can predict patient outcomes based on various factors such as age, medical history, and lifestyle choices. This predictive capability allows healthcare professionals to intervene proactively, enhancing patient care and optimizing resource allocation.
4. Streamlining Administrative Processes
Machine learning can automate administrative tasks in healthcare, such as scheduling and billing, by analyzing vast amounts of operational data. This streamlining not only saves time but also reduces human error, improving overall service delivery.
Challenges in Utilizing Medical Datasets for Machine Learning
Despite the substantial benefits, leveraging medical datasets for machine learning is not without its challenges:
1. Data Privacy and Security
Given the sensitive nature of medical information, ensuring data privacy and security is a paramount concern. Organizations must comply with regulations such as HIPAA in the United States to safeguard patient information.
2. Data Quality and Standardization
The effectiveness of machine learning algorithms hinges on the quality and consistency of the data. Inconsistent data formats and incomplete information can lead to unreliable model outputs, necessitating robust data cleaning and standardization processes.
3. Interpretability of Algorithms
Many machine learning algorithms, particularly deep learning models, can act as “black boxes,” making it challenging for healthcare professionals to understand how decisions are made. This lack of transparency can hinder trust and acceptance among practitioners.
Best Practices for Using Medical Datasets in Machine Learning
To optimize the use of medical datasets for machine learning, consider the following best practices:
1. Collaborate with Healthcare Experts
Engaging healthcare professionals during the dataset preparation and model development phases ensures that the insights generated are clinically relevant and actionable.
2. Prioritize Patient Privacy
Ensure that all data handling practices are compliant with legal and ethical standards regarding patient confidentiality. Implement stringent data access controls and anonymization techniques.
3. Conduct Thorough Data Preprocessing
Prior to training machine learning models, conduct extensive data preprocessing to rectify inconsistencies, manage missing values, and standardize formats—a critical step in enhancing model performance.
4. Foster an Iterative Learning Environment
Machine learning is not a one-time endeavor. Continuously evaluate and update models with new data to improve accuracy and adapt to changing clinical practices.
Case Studies: Successful Applications of Machine Learning in Healthcare
Numerous organizations have successfully implemented medical datasets for machine learning to achieve significant improvements in healthcare delivery. Below are compelling case studies:
1. IBM Watson Health
IBM Watson Health has harnessed the power of machine learning to analyze vast datasets, aiding clinical decision-making by providing oncologists with evidence-based treatment options tailored to individual patients. This capability has paved the way for more precise cancer treatments and improved patient outcomes.
2. Google DeepMind
DeepMind has developed machine learning algorithms that analyze eye scans to diagnose diabetic retinopathy with accuracy comparable to that of expert clinicians. This advancement facilitates early intervention, potentially saving patients from severe vision loss.
3. Tempus
Tempus utilizes artificial intelligence to analyze clinical and molecular data to enhance oncology treatment. By leveraging diverse datasets, they can help oncologists make data-driven decisions that optimize patient outcomes.
The Future of Medical Datasets for Machine Learning
The future of healthcare will undoubtedly be shaped by the continued integration of medical datasets for machine learning. As technology progresses, the potential applications of machine learning in healthcare will expand, leading to:
1. Enhanced Drug Discovery
Machine learning can significantly accelerate the drug discovery process by analyzing biological data to identify potential drug candidates and predict their efficacy before clinical trials.
2. Improved Patient Monitoring
Wearable devices and mobile health applications will continue to generate vast amounts of health data. Harnessing this data through machine learning can facilitate more effective real-time monitoring and management of chronic conditions.
3. Predictive Analytics in Public Health
Machine learning algorithms will enable public health officials to predict and mitigate outbreaks by analyzing various datasets, enhancing the responsiveness of healthcare systems to public health crises.
Conclusion
The incorporation of medical datasets for machine learning in healthcare is a game-changer, granting practitioners access to powerful tools that can improve patient care and streamline processes. While there are challenges to address, the vast potential for enhanced diagnostics, personalized medicine, and operational efficiency is undeniable. As more organizations embrace this technology, the healthcare landscape will continue to evolve, ultimately leading to better outcomes for patients everywhere.
In conclusion, the transformative role of machine learning in healthcare, underpinned by robust medical datasets, promises not only to enhance individual patient care but also to revolutionize entire healthcare systems. As we move forward, embracing innovation and data-driven approaches will be crucial in building a healthier future.
medical dataset for machine learning